How to Request a Quote
Form
Please download and fill in the
Services Registration Form.
Email
And then send the form to [email protected]
Feedback
We will contact you by email as soon as possible, please make sure your Email address is correct and check your email in time.
1. Overview
1.1 Introduction of Virtual Screening
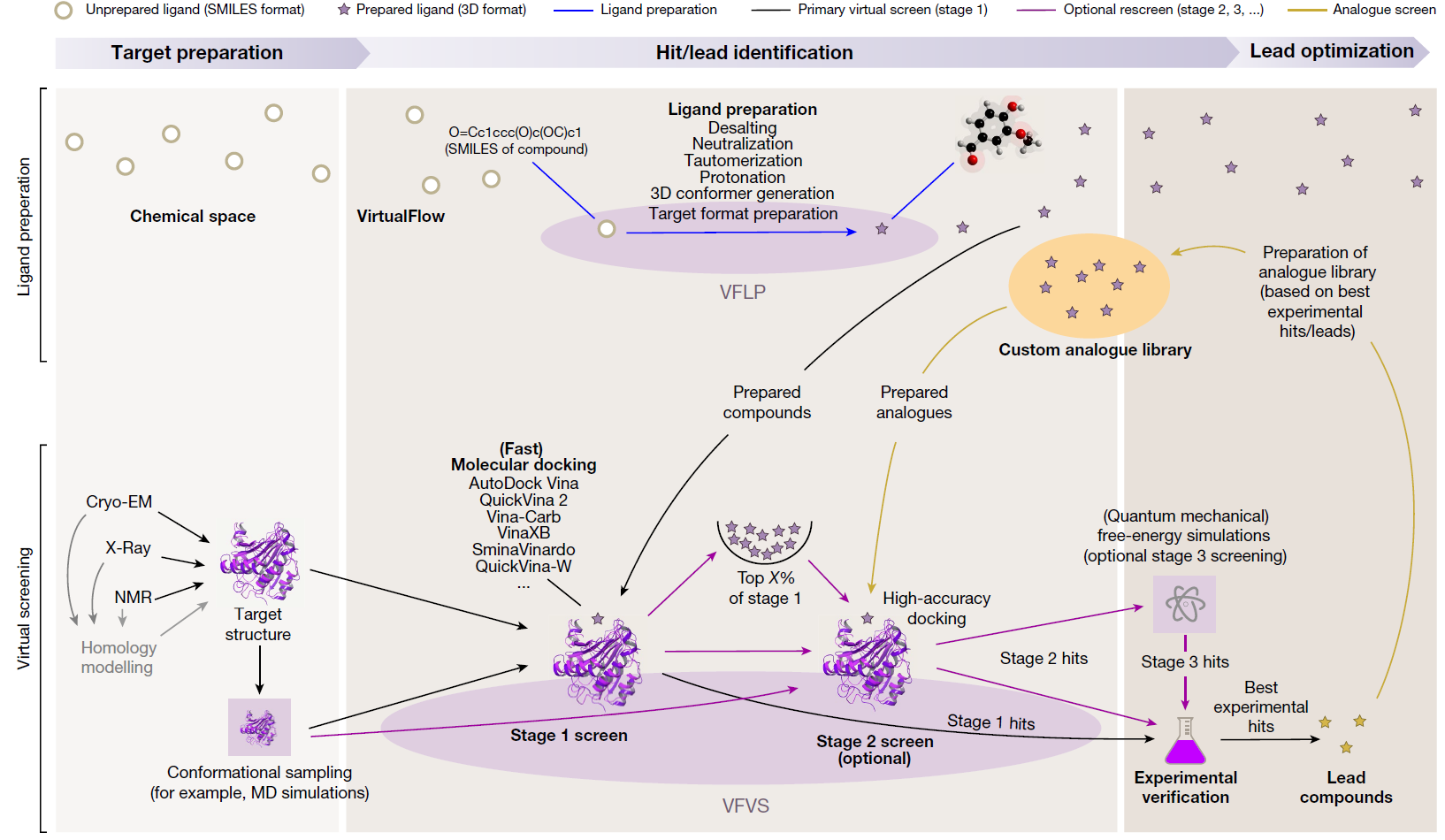
Virtual screening (VS) is a computational technique used in drug discovery to search libraries of small molecules in order to identify those structures which are most likely to bind to a drug target, typically a protein receptor or enzyme. Using the molecular docking between small molecule compounds and drug targets, VS can quickly select active compounds with druggability from tens to millions of molecules, greatly reducing the number of experimental compounds, shortening the research cycle, and reducing the cost of drug development. Virtual screening methods are mainly divided into structure-based virtual screening (SBVS) and ligand-based virtual screening (LBVS). According to reports, the positive rate of VS is 5%-30%. In nowadays, virtual screening has become the most promising drug development tool.
1.1.1 SBVS
SBVS involves docking of candidate ligands into a protein target followed by applying a scoring function to estimate the likelihood that the ligand will bind to the protein with high affinity. The SBVS approach utilizes the knowledge of the 3D structure of the biological target in the process to select ligands with acceptable affinity and complementarity with the binding site. Then, the results can be used for subsequent experiments.
1.1.2 LBVS
LBVS is the approach of choice when the biological target is not known or its 3D structure is not available. Given a set of structurally diverse ligands that binds to a receptor, a model of the receptor can be built by exploiting the collective information contained in such set of ligands. These are known as pharmacophore models. A candidate ligand can then be compared to the pharmacophore model to determine whether it is compatible with it and therefore likely to bind. The approach we used for LBVS is based on searching molecules with shape similar to that of known actives, as such molecules will fit the target\'s binding site and hence will be likely to bind the target.
2. Workflow
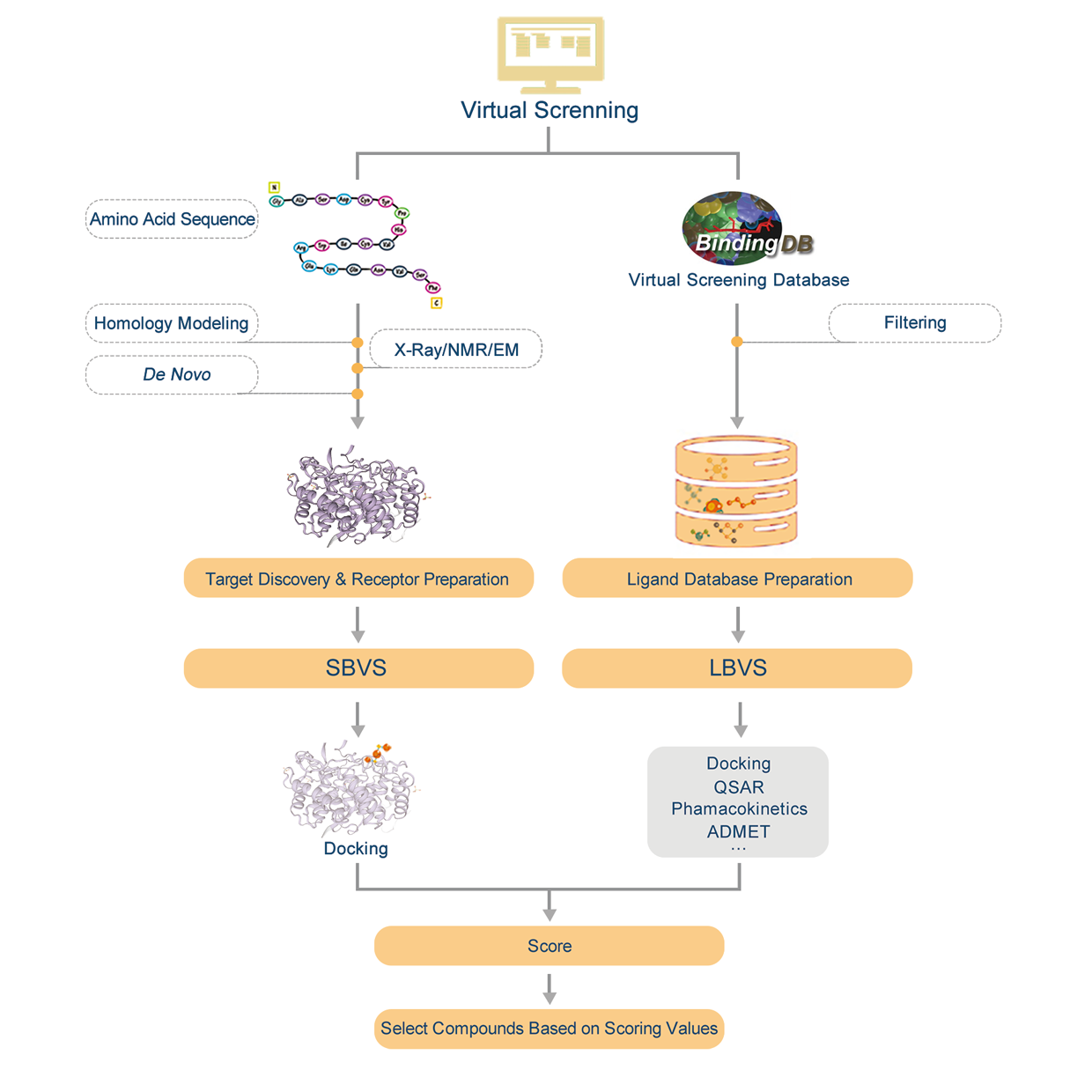
Introduction:
1. Find homologous proteins of the protein we have within the database.
2. Do homology modeling online with SWISS-MODEL and evaluate the effectiveness of modeling according by GMQE&QMEAN.
3. Download PDB file.
4. Confirm the amino acid sequence and secondary structure of proteins.
5. Predict active sites or find active sites by protein alignments.
6. Preparations of molecule docking, such as structural optimization and prepare for glide grid files.
7. Molecule docking and evaluation.
3. Applications
3.1 Pharmacophore-Based Virtual Screening Toward the Discovery of Novel Anti-echinococcal Compounds
3.1.1 Abstract
Echinococcosis is a serious helminthic zoonosis with a great impact on human health and livestock husbandry. However, the clinically used drugs (benzimidazoles) have a low cure rate, so alternative drugs are urgently needed. Currently, drug screenings for echinococcosis are mainly phenotype-based, and the efficiency of identifying active compounds is very low. With a pharmacophore model generated from the structures of active amino alcohols, researchers performed a virtual screening to discover novel compounds with anti-echinococcal activity. Sixty-two compounds from the virtual screening were tested on Echinococcus multilocularis protoscoleces, and 10 of these compounds were found to be active.
3.1.2 Results
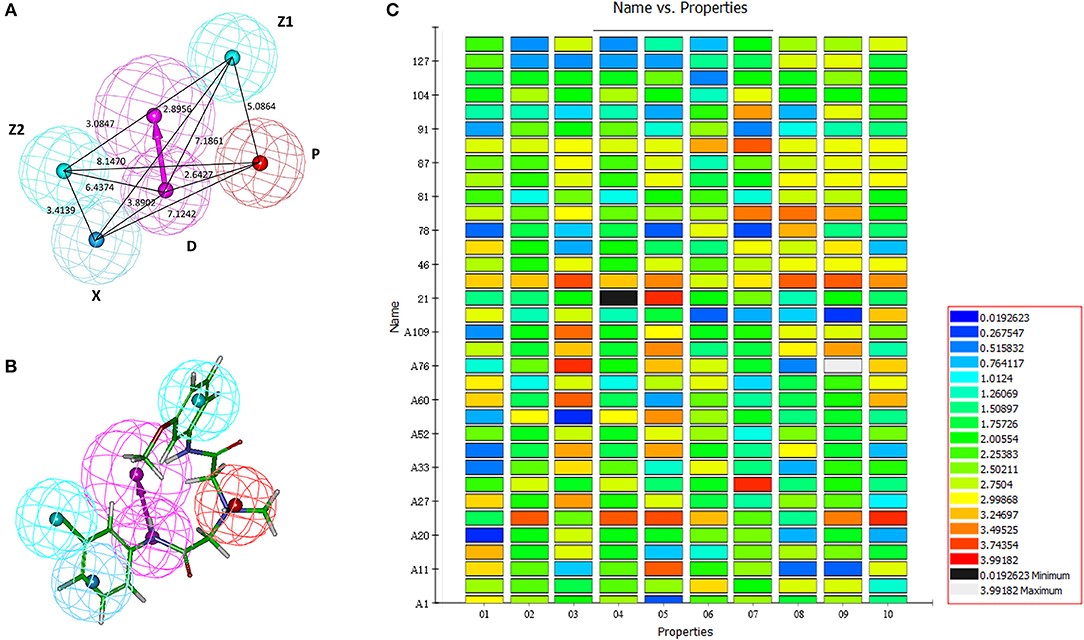
Figure 3.1.1 Generation of the HipHop pharmacophore. (A) The HipHop-Hypo03 chemical features. The color of the pharmacophore features, namely, PI, HAR, HAL and HBD, are red, blue, cyan, and magenta, respectively. (B) S6 fit to HipHop-Hypo03. (C) The heat map of the 10 hypotheses in the test.
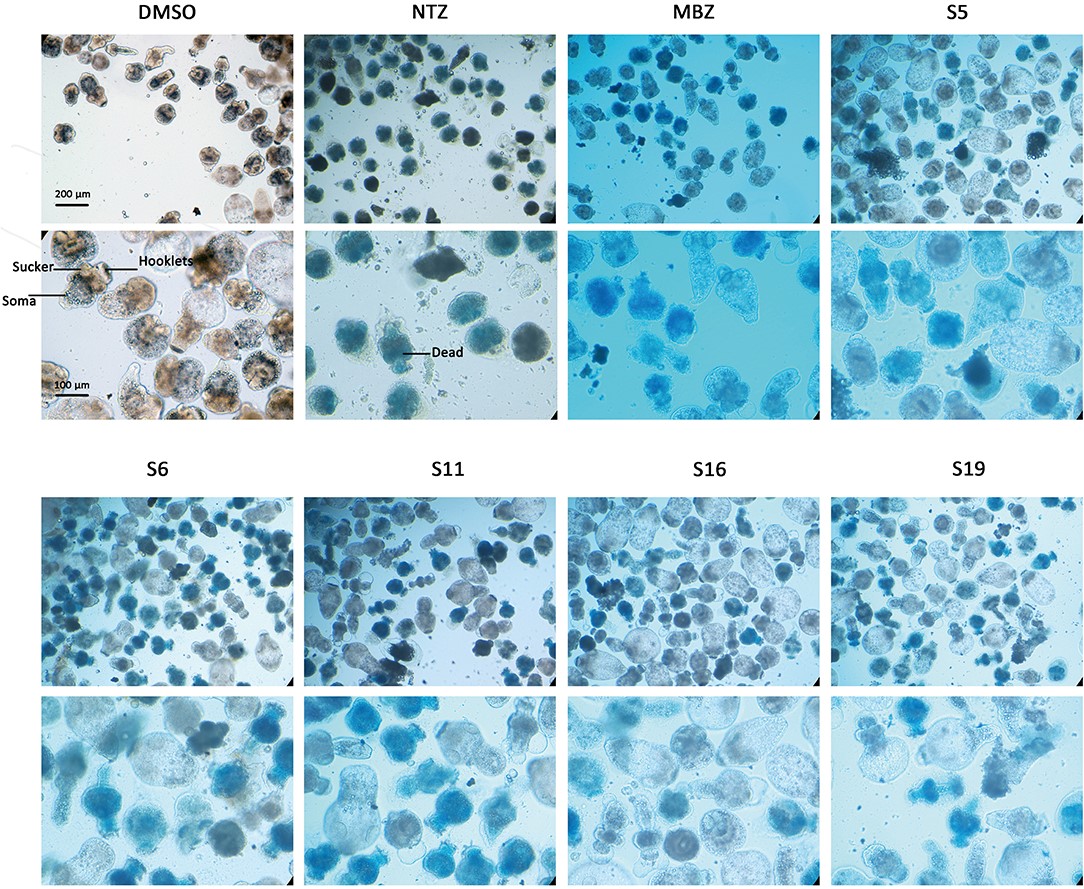
Figure 3.1.2 Morphology changes in Echinococcus multilocularis protoscoleces after incubation with the active compounds at 25 μM for 3 days. The normal protoscoleces in DMSO had intact soma, sucker and orderly arranged hooks. The drug treated parasites showed partial collapse and disruption of the inner structure, the detachment of hooks and staining with methylene blue.
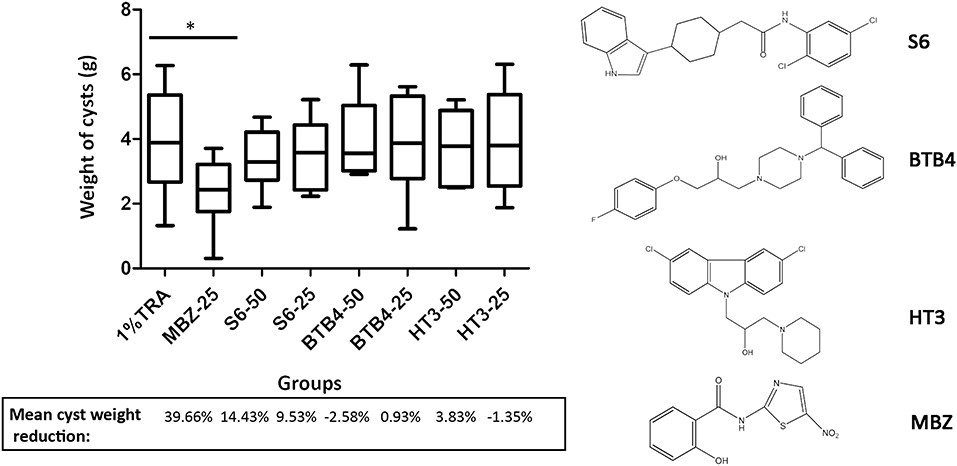
Figure 3.1.3 Treatment of mice secondarily infected with E. multilocularis. BALB/c mice were treated with S6, BTB4, and HT3 (25 mg/kg and 50 mg/kg), MBZ (25 mg/kg), or 1% TRA (control) for 28 days, n = 10. MBZ-25, BTB4-50, BTB4-25, HT3-50, and HT3-25 resulted in the deaths of 1, 4, 2, 3, and 2 mice, respectively. *p < 0.05.
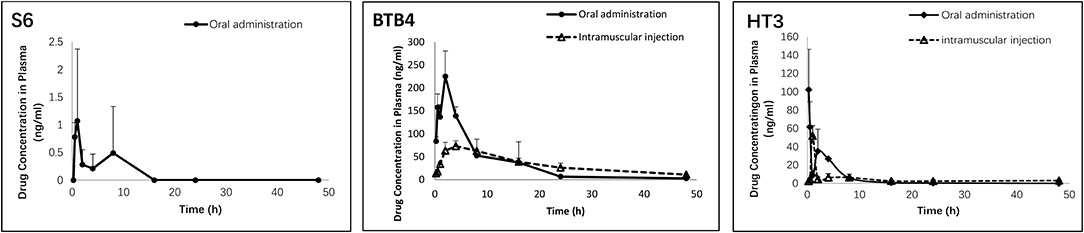
Figure 3.1.4 Pharmacokinetic parameters of S6, HT3 and BTB4 in the plasma of mice after oral (50 mg/kg) and intramuscular (12.5 mg/kg) administration.
3.2 Identification of SARS-CoV-2 Cell Entry Inhibitors by Drug Repurposing Using in silico Structure-Based Virtual Screening Approach
3.2.1 Abstract
The rapidly spreading, highly contagious and pathogenic SARS-coronavirus 2 (SARS-CoV-2) associated Coronavirus Disease 2019 (COVID-19) has been declared as a pandemic by the World Health Organization (WHO). The novel 2019 SARS-CoV-2 enters the host cell by binding of the viral surface spike glycoprotein (S-protein) to cellular angiotensin converting enzyme 2 (ACE2) receptor. The virus specific molecular interaction with the host cell represents a promising therapeutic target for identifying SARS-CoV-2 antiviral drugs. The repurposing of drugs can provide a rapid and potential cure toward exponentially expanding COVID-19. Thereto, high throughput virtual screening approach was used to investigate FDA approved LOPAC library drugs against both the receptor binding domain of spike protein (S-RBD) and ACE2 host cell receptor. Primary screening identified a few promising molecules for both the targets, which were further analyzed in details by their binding energy, binding modes through molecular docking, dynamics and simulations. Evidently, GR 127935 hydrochloride hydrate, GNF-5, RS504393, TNP, and eptifibatide acetate were found binding to virus binding motifs of ACE2 receptor. Additionally, KT203, BMS195614, KT185, RS504393, and GSK1838705A were identified to bind at the receptor binding site on the viral S-protein. These identified molecules may effectively assist in controlling the rapid spread of SARS-CoV-2 by not only potentially inhibiting the virus at entry step but are also hypothesized to act as anti-inflammatory agents, which could impart relief in lung inflammation. Timely identification and determination of an effective drug to combat and tranquilize the COVID-19 global crisis is the utmost need of hour. Further, prompt in vivo testing to validate the anti-SARS-CoV-2 inhibition efficiency by these molecules could save lives is justified.
3.2.2 Results
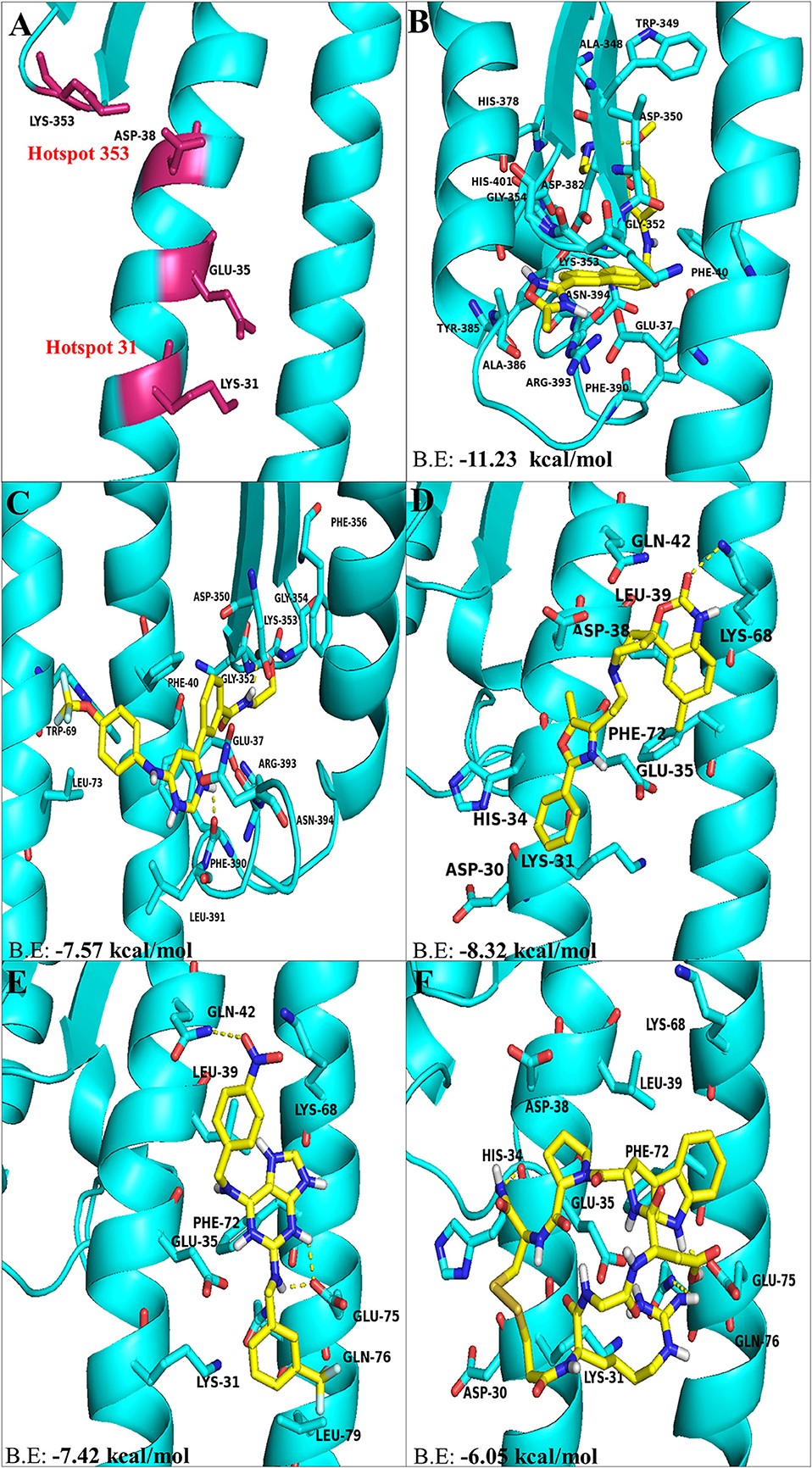
Figure 3.2.1 The top hit selected ligands from LOPAC library showing molecular interactions with ACE2 receptor of the host cell. (A) Hotspot 31 and hotspot 353 residues of ACE2 receptor responsible for recognizing S-RBD of SARS-CoV-2. (B) Molecular interactions of GR hydrochloride with ACE2 receptor. (C) Molecular interactions of GNF-5 with ACE2 receptor. (D) Molecular interactions of RS504393 with ACE2 receptor. (E) Molecular interactions of TNP with ACE2 receptor. (F) Molecular interactions of eptifibatide acetate with ACE2 receptor. Blue ribbons corresponds to residues of ACE2 receptor and yellow stick model represents residues of Ligands. BE, Binding energy.
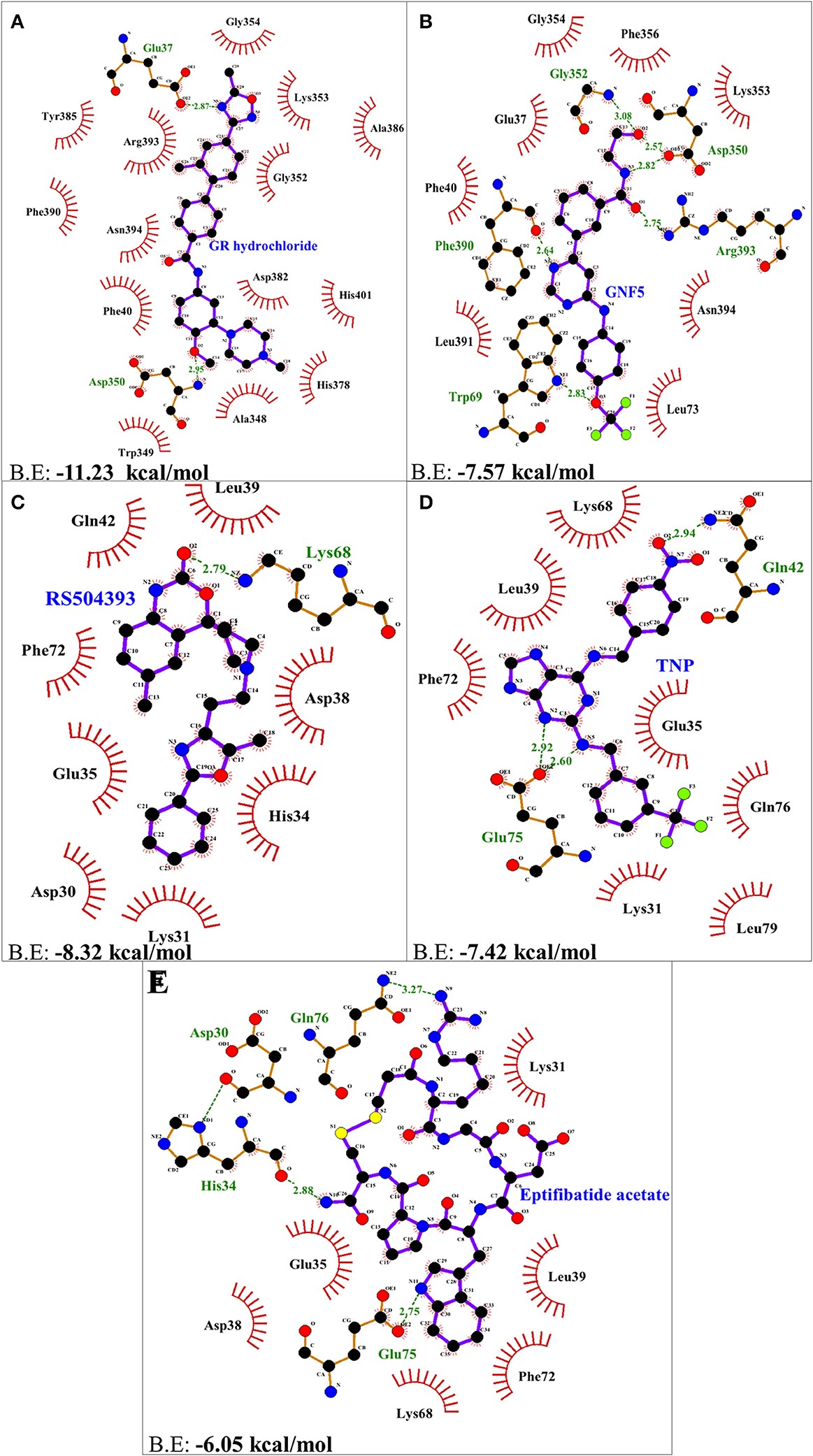
Figure 3.2.2 Two dimensional representation of H-bonds and hydrophobic interactions of selected compound with ACE2 receptor using LigPlot. (A) GR hydrochloride (B) GNF-5 (C) RS5049393 (D) TNP (E) Eptifibatide acetate. Ligands are colored and represented in purple color, H-bonds are displayed in green dotted lines, red stellations represents hydrophobic interactions, and bonds of proteins are shown in brown color.
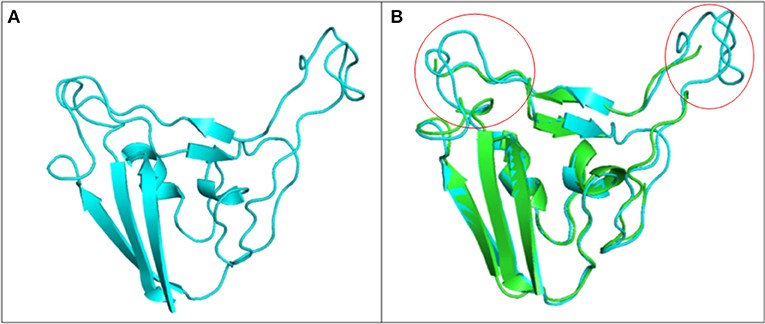
Figure 3.2.3 Structure of S1 subunit of SARS-CoV-2 (PMDB ID: PM0082972). (A) Cartoon representation of predicted S1 subunit of SARS-CoV-2. (B) Superimposition of template (PDB ID: 6VSB) and modeled S-RBD of S-protein. Predicted S-RBD and template are sky blue and green in color. Encircled area represents missing residues in loops of template S-protein which are modeled for S-RBD protein of SARS-CoV-2 using SWISS MODEL.
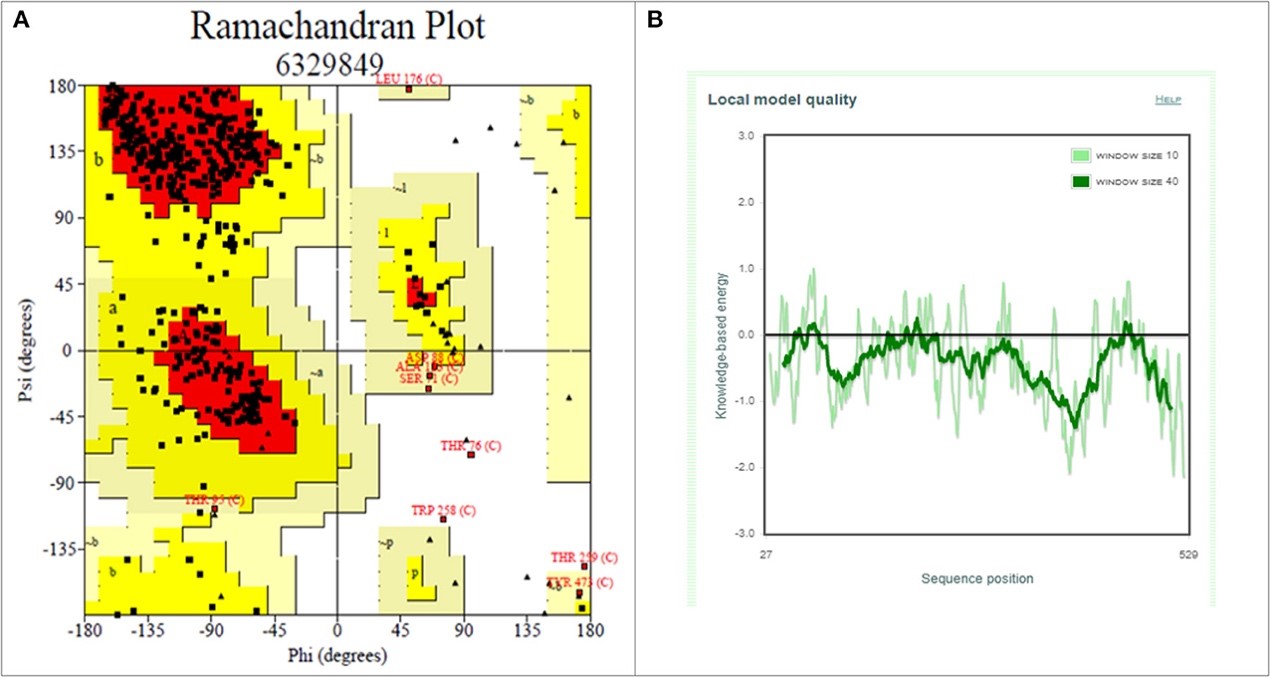
Figure 3.2.4 Structure validation of S1 subunit of S-protein by ProCheck and ProSA server. (A) ProCheck Ramachandran Plot where red, bright yellow and light yellow color represents that 99.4% residues of predicted S1 subunit of S-protein are present in favorably allowed region and 0.7% residues are present in disallowed region (lightest yellow). (B) Energy profile of modeled spike protein of SARS-CoV-2 as calculated by ProSA.
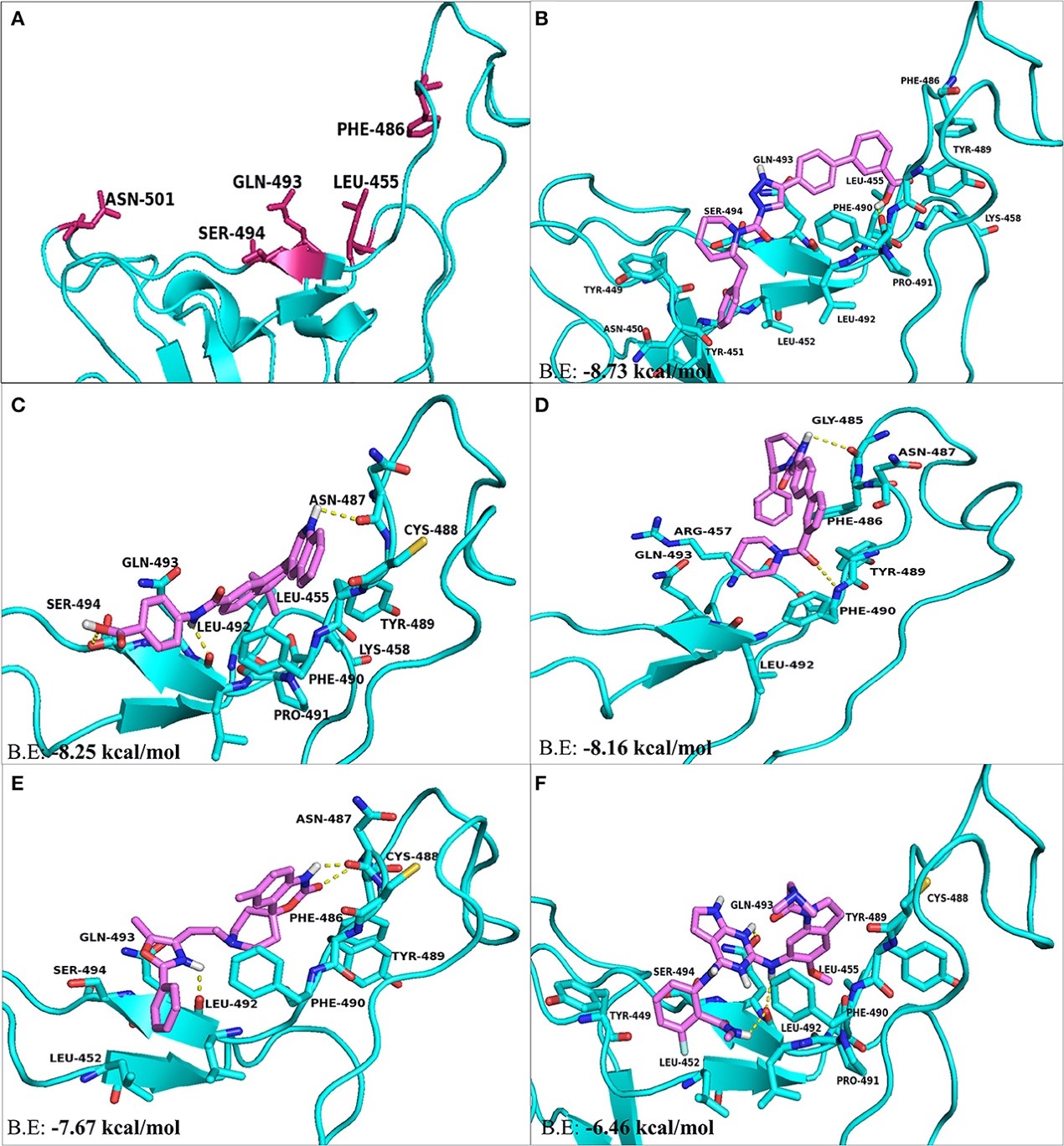
Figure 3.2.5 The top hit selected ligands from LOPAC library showing molecular interactions with S-RBD protein of SARS-CoV-2. (A) S-RBD residues responsible for interacting with ACE2 receptor. (B) Molecular interactions of KT203 with S-RBD. (C) Molecular interactions of BMS195614 with S-RBD. (D) Molecular interactions of KT185 with S-RBD. (E) Molecular interactions of RS504393 with S-RBD. (F) Molecular interactions of GSK1838705A with S-RBD. Blue ribbons corresponds S-RBD of spike protein of SARS-CoV-2 and violet stick model represents residues of Ligands.
4. References
[1] Liu C, Yin J, Yao J, Xu Z, Tao Y, Zhang H. Pharmacophore-Based Virtual Screening Toward the Discovery of Novel Anti-echinococcal Compounds. Front Cell Infect Microbiol. 2020; 10: 118. Published 2020 Mar 20. doi:10.3389/fcimb.2020.00118
[2] Choudhary S, Malik YS, Tomar S. Identification of SARS-CoV-2 Cell Entry Inhibitors by Drug Repurposing Using in silico Structure-Based Virtual Screening Approach. Front Immunol. 2020; 11: 1664. doi:10.3389/fimmu.2020.01664